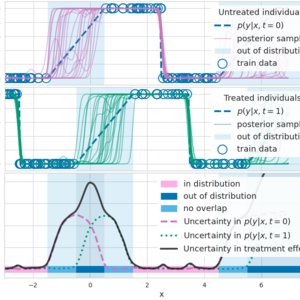
When causal inference fails - detecting violated assumptions with uncertainty-aware models - OATML
- Article
- Dec 8, 2020
- #MachineLearning #DataScience
Effective personalised treatment recommendations are enabled by knowing precisely how someone will respond to treatment. When there is sufficient knowledge about both the population...
Show More