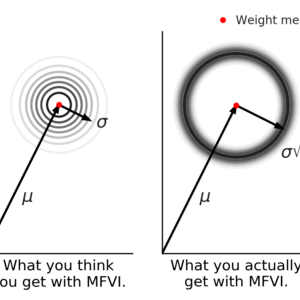
Beyond Discrete Support in Large-scale Bayesian Deep Learning - OATML
- Paper
- Apr 22, 2020
- #Deeplearning #Neuroscience #MachineLearning
Most neural networks learn a single estimate of the best network weights. But, in reality, we are unsure. What we really want is to learn a probability distribution over those weigh...
Show More